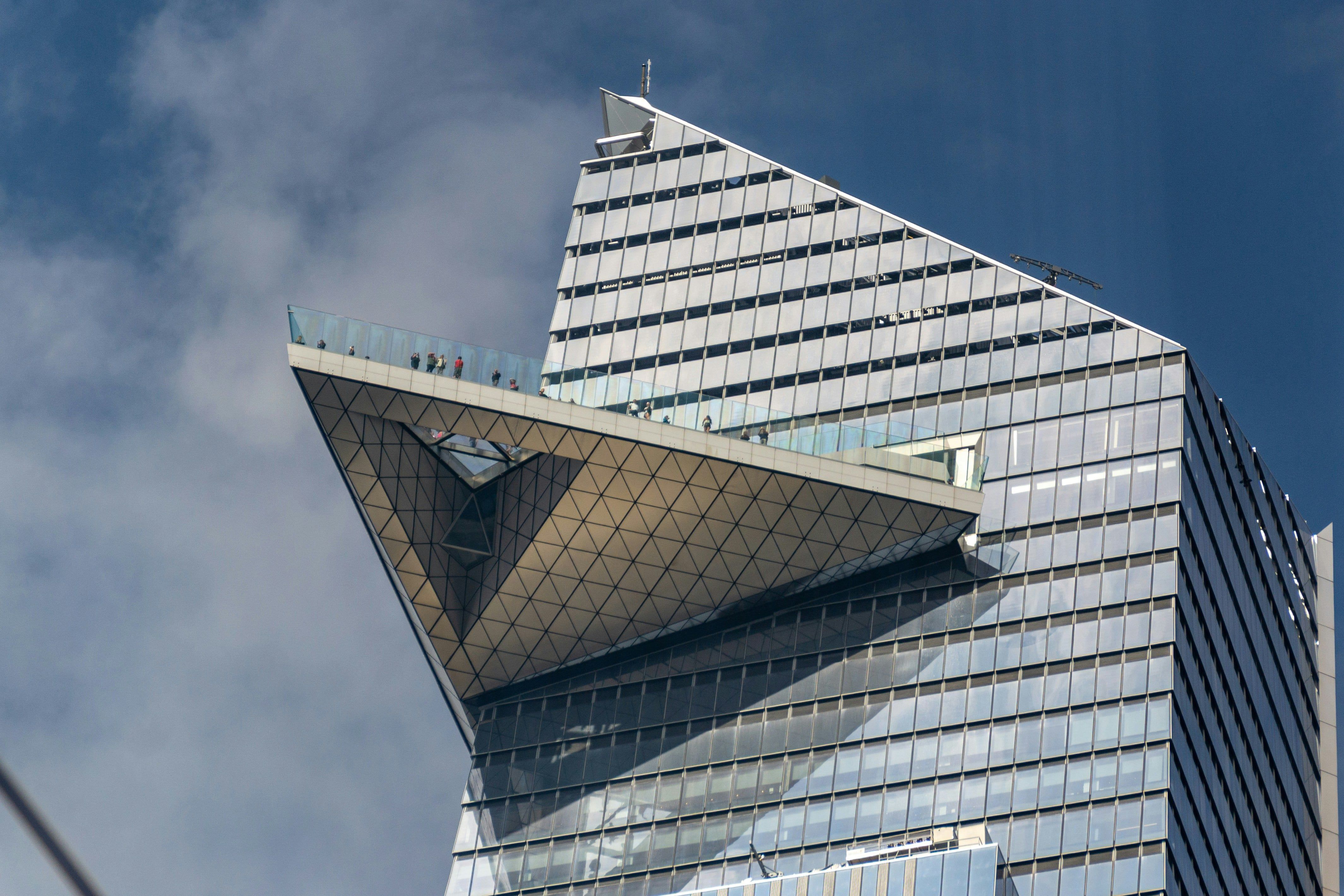
Hallucination Rate
What is Hallucination Rate?
Hallucination Rate is a metric used to evaluate the frequency at which an AI model generates outputs that are incorrect, irrelevant, or fabricated based on its training data. It is a common issue in generative AI and large language models (LLMs), where the system “hallucinates” information that may appear plausible but is factually inaccurate.
Why is it Important?
Hallucination Rate is critical for assessing the reliability and accuracy of AI systems. High hallucination rates can undermine trust in AI applications, especially in domains requiring factual accuracy, such as healthcare, finance, or legal systems. Reducing hallucination rates ensures AI-generated outputs are credible, enhancing user confidence and adoption.
How is This Metric Managed and Where is it Used?
Hallucination Rate is managed by improving model training, fine-tuning on domain-specific data, and incorporating feedback loops to correct errors. It is used in applications such as chatbots, generative AI tools, and content creation systems, where maintaining accuracy is crucial.
Key Elements
- Training Data Quality: Ensures the model learns from accurate and comprehensive datasets.
- Contextual Understanding: Improves the model’s ability to generate relevant and factual outputs.
- Feedback Mechanisms: Incorporates user feedback to identify and reduce hallucinations.
- Domain-Specific Fine-Tuning: Customizes the model for accuracy in specialized fields.
- Evaluation Metrics: Regularly assesses outputs for accuracy and relevance.
Recent Posts
Real-World Examples
- Chatbots: Identifying when a chatbot provides incorrect responses to user queries.
- Healthcare AI: Reducing hallucinations in medical diagnoses generated by AI systems.
- Content Generation: Ensuring articles or reports created by generative AI are factually accurate.
- Search Engines: Evaluating the correctness of AI-summarized search results.
- Customer Support: Minimizing hallucinated answers in AI-driven support systems to maintain user trust.
Use Cases
- Customer Service: Enhances the reliability of AI assistants by reducing fabricated responses.
- Content Creation Tools: Ensures outputs from generative AI tools align with factual information.
- Healthcare Diagnostics: Improves the trustworthiness of AI-generated medical insights.
- Search and Recommendation Systems: Delivers accurate and contextually relevant suggestions.
- Education Platforms: Provides factually correct and comprehensive responses for learners.
Frequently Asked Questions (FAQs):
It is the frequency at which AI models produce incorrect, irrelevant, or fabricated outputs.
It impacts the reliability and trustworthiness of AI systems, especially in critical applications like healthcare and finance.
By improving training data quality, incorporating feedback mechanisms, and fine-tuning the model for specific domains.
Industries like healthcare, finance, legal, and education are significantly impacted, where factual accuracy is vital.
AI evaluation frameworks and tools like human-in-the-loop feedback systems, error tracking, and domain-specific validators are commonly used.
Are You Ready to Make AI Work for You?
Simplify your AI journey with solutions that integrate seamlessly, empower your teams, and deliver real results. Jyn turns complexity into a clear path to success.