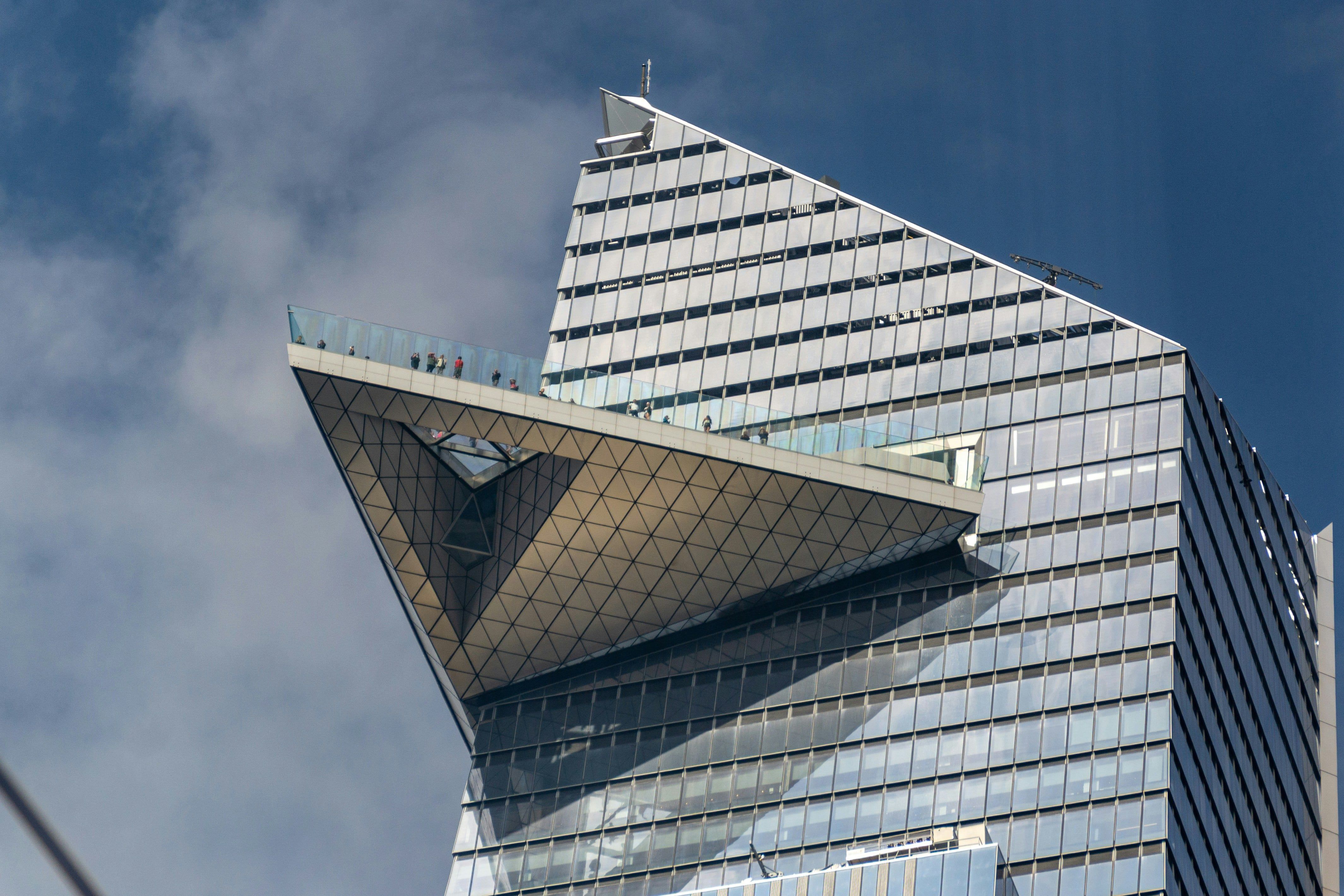
Conversational AI
What is Predictive Analytics?
Predictive Analytics is the process of using statistical models, machine learning, and data mining techniques to analyze historical data and predict future outcomes. It helps businesses and organizations make informed decisions by identifying patterns, trends, and potential risks.
Why is it Important?
Predictive Analytics enables organizations to anticipate future events, optimize operations, and improve decision-making. By leveraging data-driven insights, businesses can reduce risks, enhance customer experiences, and achieve competitive advantages in dynamic markets.
How is This Metric Managed and Where is it Used?
Predictive Analytics relies on data preparation, model selection, and evaluation. Key steps include cleaning data, applying algorithms like regression and decision trees, and validating model accuracy. It is widely used in industries like finance (credit scoring), healthcare (patient risk prediction), marketing (customer segmentation), and supply chain management (demand forecasting).
Key Elements
- Data Collection: Gathering historical and real-time data for analysis.
- Statistical Modeling: Applying methods like regression analysis to understand trends.
- Machine Learning Algorithms: Leveraging supervised and unsupervised learning for prediction.
- Visualization Tools: Presenting predictions through dashboards and reports.
- Model Validation: Testing and refining models for better accuracy and reliability.
Recent Posts
Real-World Examples
- Credit Scoring: Banks and financial institutions use predictive models to evaluate a borrower’s creditworthiness, identifying the likelihood of defaults or delinquencies based on historical transaction data and behavioral patterns.
- Healthcare Risk Analysis: Predictive analytics tools analyze patient data, including medical history and lifestyle factors, to flag individuals at high risk for chronic diseases, enabling preventive care and reducing long-term healthcare costs.
- Churn Prediction: Subscription-based businesses like streaming services or telecom providers leverage predictive models to identify at-risk customers and implement retention strategies, such as offering personalized incentives.
- Demand Forecasting: Retailers and manufacturers use demand forecasting models to predict product demand based on seasonality, sales trends, and external factors like weather, ensuring optimal inventory levels and reduced wastage.
- Fraud Detection: Financial systems utilize predictive algorithms to detect unusual transaction patterns in real-time, helping to identify fraudulent activities such as identity theft or phishing scams before they cause significant harm.
- Predictive Maintenance: Industries like manufacturing and aviation employ predictive analytics to monitor equipment health and predict failures, minimizing unplanned downtime and repair costs.
- Personalized Education: EdTech platforms use predictive analytics to tailor learning experiences based on student performance, identifying those who may need additional support.
Use Cases
- Marketing: Businesses analyze consumer behavior and purchase history to predict future buying patterns, enabling hyper-personalized marketing campaigns and enhancing customer engagement.
- Supply Chain: Predictive analytics optimizes supply chain workflows by forecasting demand spikes, identifying potential bottlenecks, and suggesting alternative logistics routes to avoid delays.
- Retail: Brick-and-mortar and e-commerce platforms use predictive pricing models to adjust prices dynamically, offering competitive pricing while maximizing profitability.
- Healthcare: Hospitals leverage predictive tools to forecast patient admissions and resource requirements, ensuring adequate staffing and equipment availability.
- Energy: Predictive analytics assists in forecasting energy consumption patterns, optimizing energy grid management, and predicting failures in critical infrastructure like power plants.
- Human Resources: Companies predict employee attrition using historical HR data, enabling proactive measures like career development plans or personalized engagement strategies.
- Public Safety: Government agencies analyze crime trends to predict and prevent incidents, allocating resources effectively for law enforcement.
Frequently Asked Questions (FAQs)
It uses statistical techniques and machine learning to analyze historical data and predict future trends.
Industries like healthcare, finance, retail, and logistics heavily use Predictive Analytics.
Machine learning automates the creation of predictive models, improving accuracy and scalability.
When backed by quality data and robust models, it delivers reliable and actionable insights.
Predictive Analytics focuses on forecasting specific outcomes, while AI encompasses broader decision-making and automation capabilities.
Are You Ready to Make AI Work for You?
Simplify your AI journey with solutions that integrate seamlessly, empower your teams, and deliver real results. Jyn turns complexity into a clear path to success.