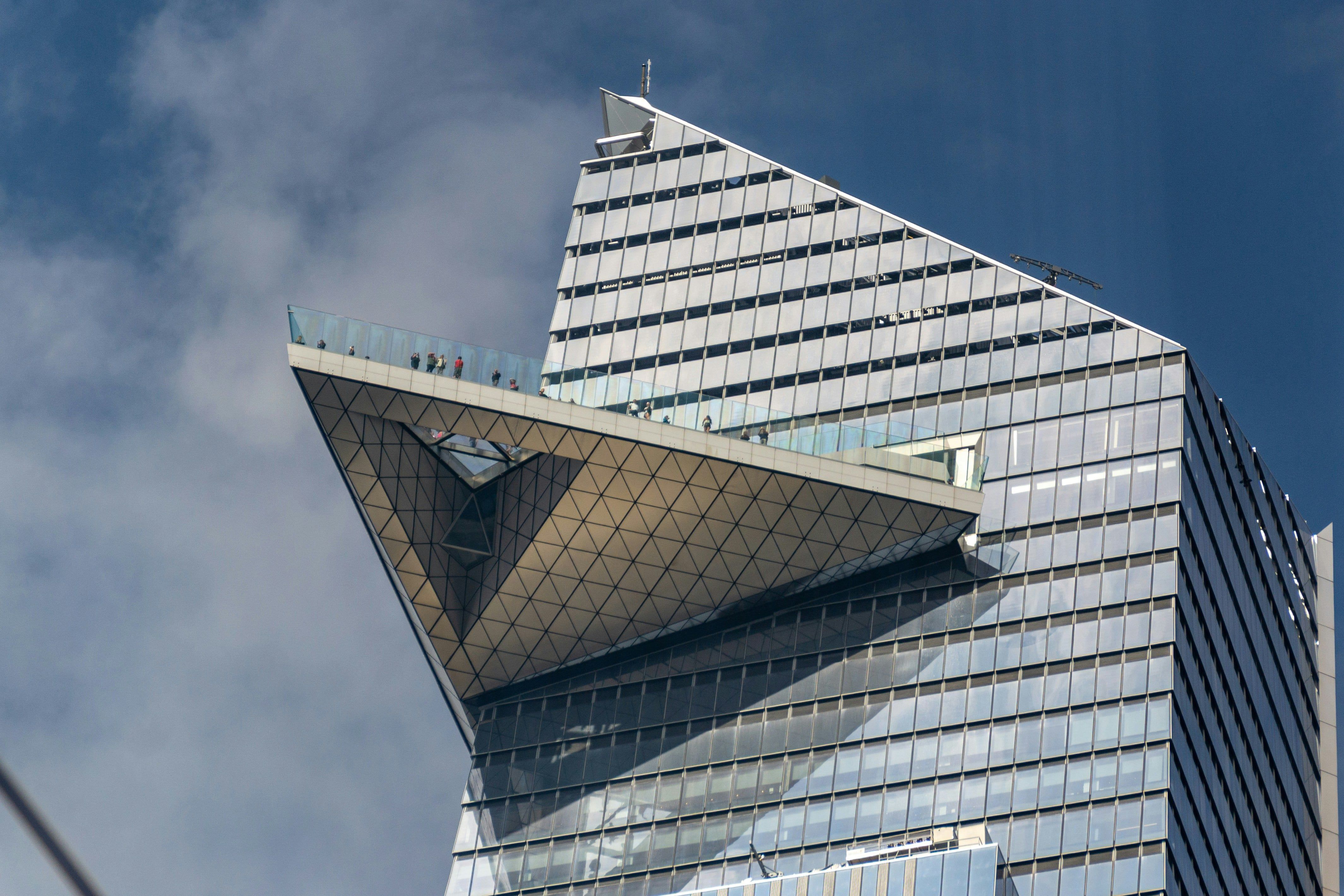
Explainable AI (XAI)
What is Explainable AI (XAI)?
Explainable AI (XAI) refers to a set of tools and frameworks designed to make artificial intelligence models more transparent and interpretable. XAI aims to provide clear explanations of how AI systems make decisions, enabling users to understand and trust their outputs while identifying and mitigating potential biases.
Why is it Important?
Explainable AI is critical for fostering trust and accountability in AI systems, especially in sensitive domains like healthcare, finance, and legal services. By making AI models more interpretable, XAI ensures compliance with regulations, reduces biases, and enhances user confidence in AI-driven decisions.
How is This Metric Managed and Where is it Used?
XAI is managed by using interpretable models, visualization tools, and explanation techniques like SHAP (SHapley Additive exPlanations) and LIME (Local Interpretable Model-agnostic Explanations). It is widely used in sectors like healthcare, finance, education, and autonomous systems to explain predictions and refine AI applications.
Key Elements
- Transparency: Provides insights into the internal workings of AI models.
- Bias Detection: Identifies and mitigates potential biases in AI outputs.
- User Trust: Builds confidence by offering clear explanations of decisions.
- Regulatory Compliance: Ensures AI systems meet legal standards for interpretability.
- Model Debugging: Helps developers identify and fix errors in AI systems.
Recent Posts
Real-World Examples
- Healthcare Diagnostics: Explains AI-driven diagnoses and treatment recommendations to doctors and patients.
- Financial Risk Assessment: Clarifies credit scoring and loan approval processes in banking.
- Autonomous Vehicles: Describes the decision-making process behind actions like braking or steering.
- E-commerce Recommendations: Provides transparency into why specific products are recommended to users.
- Fraud Detection: Highlights the reasoning behind flagging transactions as fraudulent.
Use Cases
- Ethical AI Development: Ensures fairness and accountability in AI systems.
- Customer Support Tools: Increases user satisfaction by explaining chatbot or AI assistant decisions.
- Compliance in Regulated Industries: Meets legal requirements for transparency in finance, healthcare, and legal AI applications.
- Model Optimization: Identifies areas for improvement by analyzing model behavior.
- AI Training and Adoption: Simplifies complex AI concepts for better stakeholder understanding.
Frequently Asked Questions (FAQs):
Explainable AI (XAI) refers to methods and tools that make AI models transparent and interpretable for users and stakeholders.
It fosters trust, ensures regulatory compliance, and enhances user confidence by explaining how AI systems make decisions.
XAI identifies and highlights potential biases in AI outputs, enabling developers to take corrective actions.
Industries like healthcare, finance, legal services, and autonomous systems rely on XAI for accountability and transparency.
Yes, many Conversational AI platforms support multilingual capabilities to engage users in their preferred languages.
Are You Ready to Make AI Work for You?
Simplify your AI journey with solutions that integrate seamlessly, empower your teams, and deliver real results. Jyn turns complexity into a clear path to success.