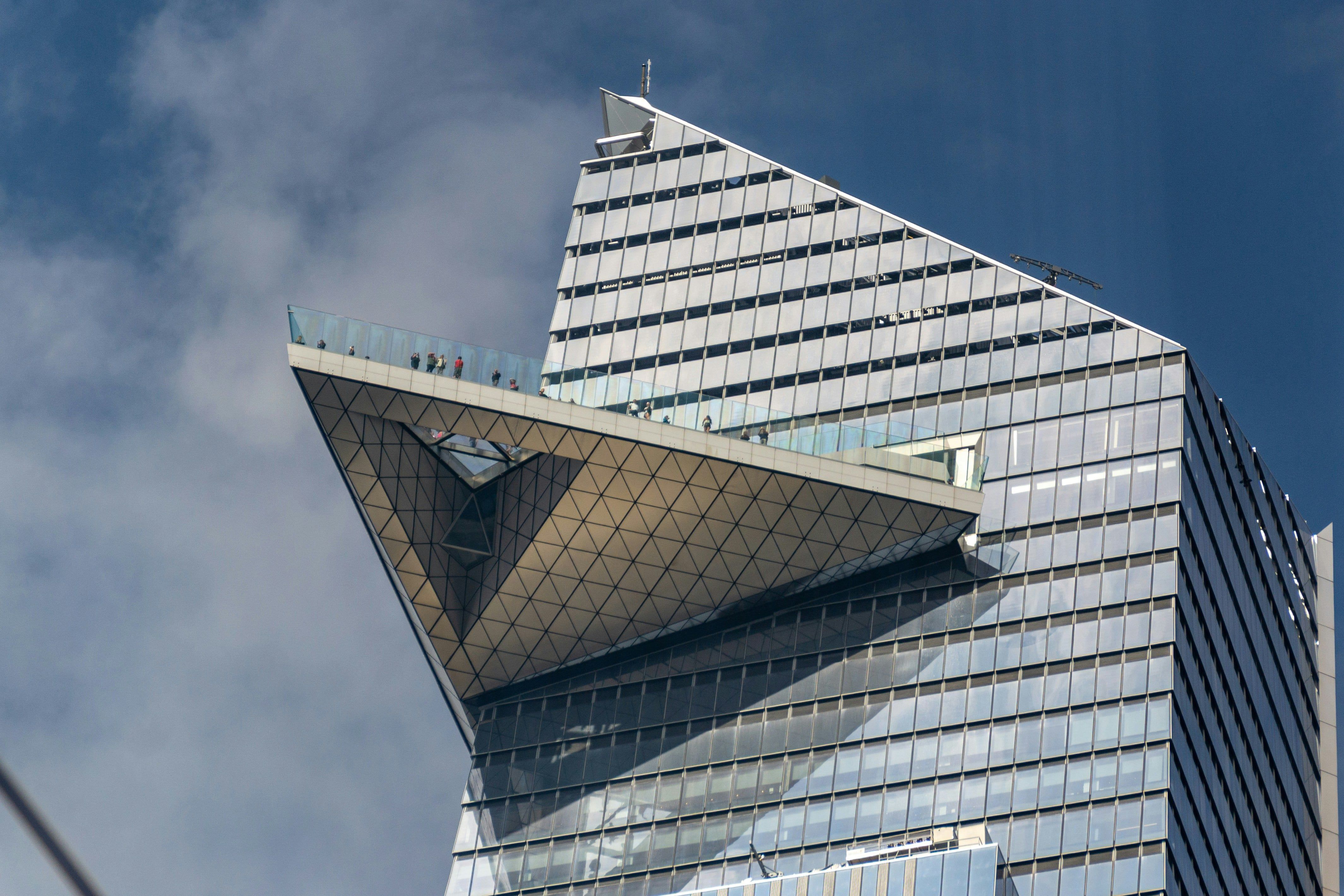
Transparency
What is Transparency?
Transparency in artificial intelligence refers to the clarity and openness with which AI systems, algorithms, and decision-making processes are designed, developed, and deployed. It ensures that users and stakeholders can understand how AI systems work, how decisions are made, and what data influences these outcomes.
Why is it Important?
Transparency is critical for building trust, ensuring accountability, and addressing ethical concerns in AI. It helps mitigate biases, comply with regulations, and improve user confidence by providing clear insights into AI processes. Transparent AI systems are crucial for sensitive domains like healthcare, finance, and legal services.
How is This Metric Managed and Where is it Used?
Transparency is achieved through practices like explainable AI (XAI), algorithm documentation, and data provenance tracking. It is widely used in AI applications where trust and accountability are essential, including automated decision-making systems, regulatory compliance tools, and public-facing AI products.
Key Elements
- Explainability: Enables users to understand how AI models generate results.
- Data Provenance: Tracks the origin, quality, and use of data in AI systems.
- Algorithm Documentation: Provides clear descriptions of model behavior and assumptions.
- Bias Mitigation: Identifies and reduces biases in training and decision-making processes.
- Ethical Standards: Ensures AI aligns with societal and organizational values.
Real-World Examples
- Healthcare Diagnostics: Explains AI-driven diagnoses and recommendations to patients and doctors.
- Financial Decision Systems: Clarifies loan approvals or credit scoring processes for customers.
- Legal AI Tools: Provides transparency in how legal documents are analyzed and recommendations made.
- Public Sector AI: Ensures fairness and accountability in AI systems for public administration.
- Retail Analytics: Offers insights into how personalized recommendations are generated for users.
Use Cases
- Regulatory Compliance: Ensures AI systems adhere to laws like GDPR by providing transparency in data usage.
- Trust Building: Enhances user confidence in AI-driven products through clear explanations.
- Bias Reduction: Identifies and mitigates potential biases in data and algorithms.
- Model Debugging: Helps developers understand and improve AI performance.
- User Education: Informs users about AI capabilities and limitations to promote responsible use.
Frequently Asked Questions (FAQs):
Transparency refers to the clarity and openness in how AI systems function, including their decision-making processes and data usage.
It fosters trust, ensures accountability, mitigates biases, and complies with ethical and legal standards.
Through practices like explainable AI, data provenance tracking, and detailed algorithm documentation.
Industries like healthcare, finance, legal services, public administration, and retail benefit from transparent AI systems.
By identifying and explaining potential biases in data or algorithms, enabling corrective measures to ensure fairness.
Are You Ready to Make AI Work for You?
Simplify your AI journey with solutions that integrate seamlessly, empower your teams, and deliver real results. Jyn turns complexity into a clear path to success.