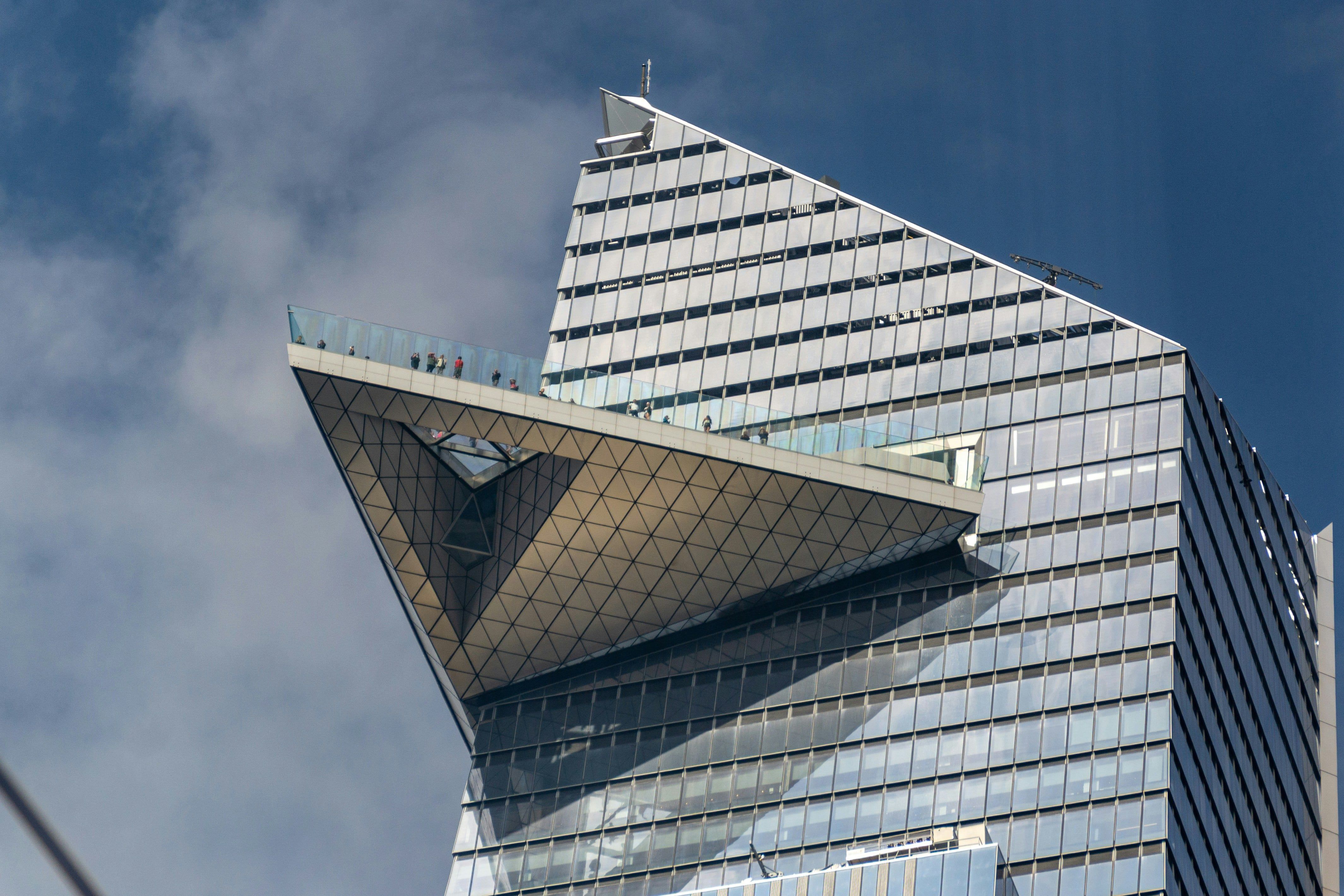
Knowledge Graph Integration
What is Knowledge Graph Integration?
Knowledge Graph Integration involves the process of connecting and incorporating knowledge graphs into AI systems, databases, or applications to enable intelligent data representation and decision-making. Knowledge graphs are structured representations of information, linking entities and their relationships to create a semantic understanding of data.
Why is it Important?
Knowledge Graph Integration is essential for enriching AI systems with semantic context, improving data retrieval, and enabling advanced reasoning capabilities. It empowers applications to understand and leverage the connections between entities, providing deeper insights and more accurate responses in domains like search engines, recommendation systems, and customer support.
How is This Metric Managed and Where is it Used?
Knowledge Graph Integration is managed by aligning data sources, standardizing schemas, and ensuring seamless connectivity between systems. It is widely used in search engines, digital assistants, e-commerce platforms, and enterprise knowledge management systems to enhance user experience and data utility.
Key Elements
- Entity Linking: Connects entities in data to their counterparts in the knowledge graph.
- Semantic Relationships: Represents how entities are connected, enabling contextual understanding.
- Interoperability: Ensures seamless integration with existing databases and systems.
- Dynamic Updates: Keeps the graph updated with real-time changes in data.
- Scalability: Accommodates growing datasets and complex relationships.
Recent Posts
Real-World Examples
- Search Engines: Enhances search results by providing entity-rich information and contextual answers.
- Virtual Assistants: Uses knowledge graphs to deliver accurate, context-aware responses.
- Recommendation Systems: Connects user preferences to relevant content or products through semantic links.
- Healthcare Applications: Links medical entities like symptoms, treatments, and conditions for better diagnostics.
- Enterprise Knowledge Management: Integrates organizational data for efficient knowledge retrieval and sharing.
Use Cases
- Semantic Search: Improves the accuracy and relevance of search engine queries.
- Content Personalization: Tailors recommendations based on user preferences and linked entities.
- Customer Support: Provides intelligent, context-aware responses by linking queries to relevant data.
- Data Analytics: Enables advanced reasoning and insights by linking structured and unstructured data.
- Knowledge Sharing: Enhances collaboration in enterprises by integrating diverse data sources.
Frequently Asked Questions (FAQs):
It is the process of incorporating knowledge graphs into AI systems or applications to enhance data connectivity and contextual understanding.
It enables applications to understand relationships between entities, improving insights, accuracy, and user experience.
By aligning data schemas, linking entities, and ensuring interoperability with existing databases and applications.
Industries like healthcare, e-commerce, education, and enterprise management use knowledge graphs to enhance decision-making and system intelligence.
Yes, many Conversational AI platforms support multilingual capabilities to engage users in their preferred languages.
Are You Ready to Make AI Work for You?
Simplify your AI journey with solutions that integrate seamlessly, empower your teams, and deliver real results. Jyn turns complexity into a clear path to success.