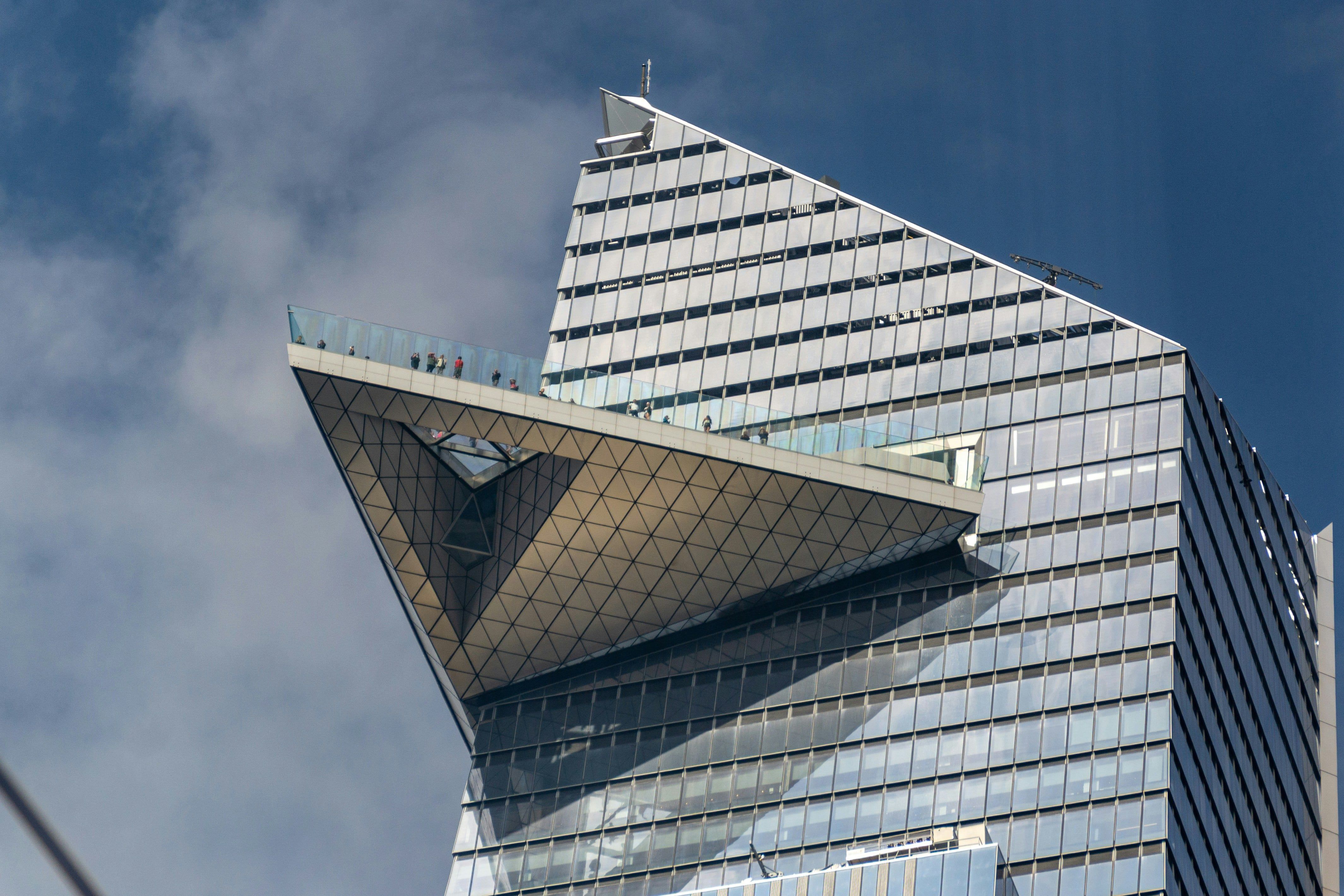
Reinforcement Learning with Human Feedback (RLHF)
What is Reinforcement Learning with Human Feedback (RLHF)?
Reinforcement Learning with Human Feedback (RLHF) is a machine learning approach where human feedback is used to guide and improve the learning process of reinforcement learning (RL) agents. By incorporating human preferences into the reward signals, RLHF ensures that AI systems align better with human expectations and ethical considerations.
Why is it Important?
RLHF enhances AI systems by making them more interpretable, reliable, and aligned with human values. It is particularly important in scenarios where traditional reward functions are insufficient to capture nuanced human preferences or when safety and ethical considerations are critical.
How is This Metric Managed and Where is it Used?
RLHF involves collecting human feedback through direct interaction or predefined guidelines and integrating it into the reinforcement learning loop. This approach is widely used in areas like conversational AI, robotics, and content moderation to align AI systems with human expectations.
Key Elements
- Human Feedback Collection: Gathers preferences or evaluations from human experts or users.
- Reward Modeling: Uses feedback to shape the reward function guiding the RL agent.
- Policy Optimization: Adjusts the AI’s behavior based on the refined reward signals.
- Iterative Improvement: Continuously incorporates feedback to refine AI performance.
- Ethical Alignment: Ensures AI behavior adheres to human-defined ethical and social norms.
Real-World Examples
- Chatbots: Improves conversational agents by aligning responses with user preferences.
- Content Moderation: Trains AI to flag inappropriate content based on human guidelines.
- Game Development: Creates NPC behaviors that align with player expectations and gameplay strategies.
- Autonomous Vehicles: Optimizes driving decisions with human feedback on safety and comfort.
- Healthcare: Aligns diagnostic AI systems with medical experts’ judgments and preferences.
Use Cases
- Conversational AI: Refines chatbot responses for natural and contextually appropriate interactions.
- Ethical AI Development: Ensures AI systems operate within socially and ethically acceptable boundaries.
- Human-Robot Interaction: Enhances robot behaviors to align with user intentions and safety norms.
- Custom AI Solutions: Personalizes AI behavior based on specific user or organizational needs.
- Policy Learning: Develops AI strategies that adhere to predefined human goals and constraints.
Frequently Asked Questions (FAQs):
RLHF is a learning approach that incorporates human feedback into the reinforcement learning process to align AI behavior with human preferences.
It enhances the reliability, interpretability, and ethical alignment of AI systems, ensuring they operate in accordance with human expectations.
Human feedback is collected and used to shape the reward signals that guide the RL agent, iteratively refining its behavior.
Industries like conversational AI, healthcare, robotics, and gaming use RLHF to improve AI alignment with human goals.
Yes, many Conversational AI platforms support multilingual capabilities to engage users in their preferred languages.
Are You Ready to Make AI Work for You?
Simplify your AI journey with solutions that integrate seamlessly, empower your teams, and deliver real results. Jyn turns complexity into a clear path to success.