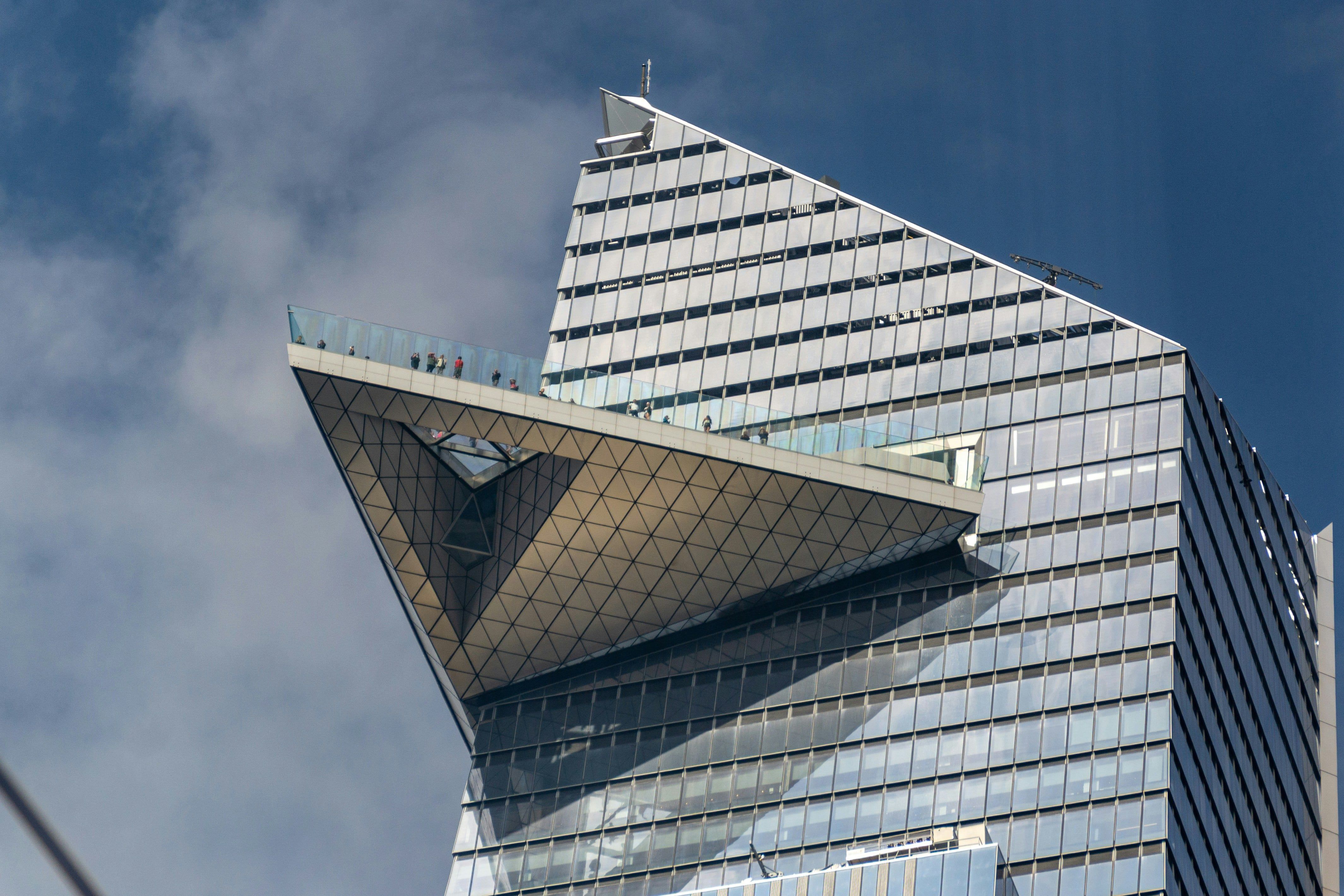
Knowledge-Enhanced Language Models
What are Knowledge-Enhanced Language Models?
Knowledge-Enhanced Language Models (KELMs) are advanced AI models that integrate external knowledge sources, such as knowledge graphs or structured databases, with traditional language models. This combination enhances the model’s ability to generate, comprehend, and reason about text by incorporating factual and contextual information.
Why is it Important?
KELMs bridge the gap between statistical language models and knowledge-based systems, enabling more accurate and context-aware text generation. They reduce hallucination (false information) and improve performance in tasks requiring factual consistency, making them critical for applications like conversational AI, education, and content generation.
How is This Metric Managed and Where is it Used?
KELMs are managed by embedding external knowledge into the model’s training and inference processes. Techniques like knowledge graph embeddings, retrieval-augmented generation, and fine-tuning with structured data are commonly used. These models find applications in industries like healthcare, education, and finance for tasks requiring high levels of accuracy and reliability.
Key Elements
- Knowledge Integration: Embeds external factual information from sources like knowledge graphs.
- Factual Consistency: Ensures generated text aligns with verified knowledge.
- Reasoning Capabilities: Supports complex reasoning tasks by leveraging structured data.
- Context Awareness: Enhances understanding of nuanced and domain-specific text.
- Multimodal Applications: Integrates knowledge across text, image, and audio inputs.
Recent Posts
Real-World Examples
- Chatbots: Provides accurate responses by integrating domain-specific knowledge bases.
- Healthcare Applications: Generates precise medical advice and explanations using structured health data.
- Search Engines: Enhances query understanding and result relevance with knowledge augmentation.
- Educational Tools: Assists in creating accurate and engaging learning materials.
- Financial Analysis: Summarizes and interprets market data with context-aware insights.
Use Cases
- Conversational AI: Enables more informative and contextually accurate dialogues.
- Content Generation: Produces factually consistent articles, summaries, and reports.
- Domain-Specific AI: Powers specialized applications in medicine, law, and engineering.
- Recommendation Systems: Improves personalization by integrating user data with external knowledge.
- Data Analysis Tools: Enhances interpretation of structured and unstructured datasets.
Frequently Asked Questions (FAQs):
Knowledge-Enhanced Language Models are AI systems that incorporate external knowledge sources to improve text understanding and generation.
They enhance accuracy, factual consistency, and context awareness in AI applications, making them essential for reliable and domain-specific tasks.
These models integrate structured knowledge, such as knowledge graphs, into their training and inference processes to produce more accurate and informed outputs.
Industries like healthcare, education, finance, and technology leverage these models for tasks requiring contextual accuracy and reasoning.
Yes, many Conversational AI platforms support multilingual capabilities to engage users in their preferred languages.
Are You Ready to Make AI Work for You?
Simplify your AI journey with solutions that integrate seamlessly, empower your teams, and deliver real results. Jyn turns complexity into a clear path to success.