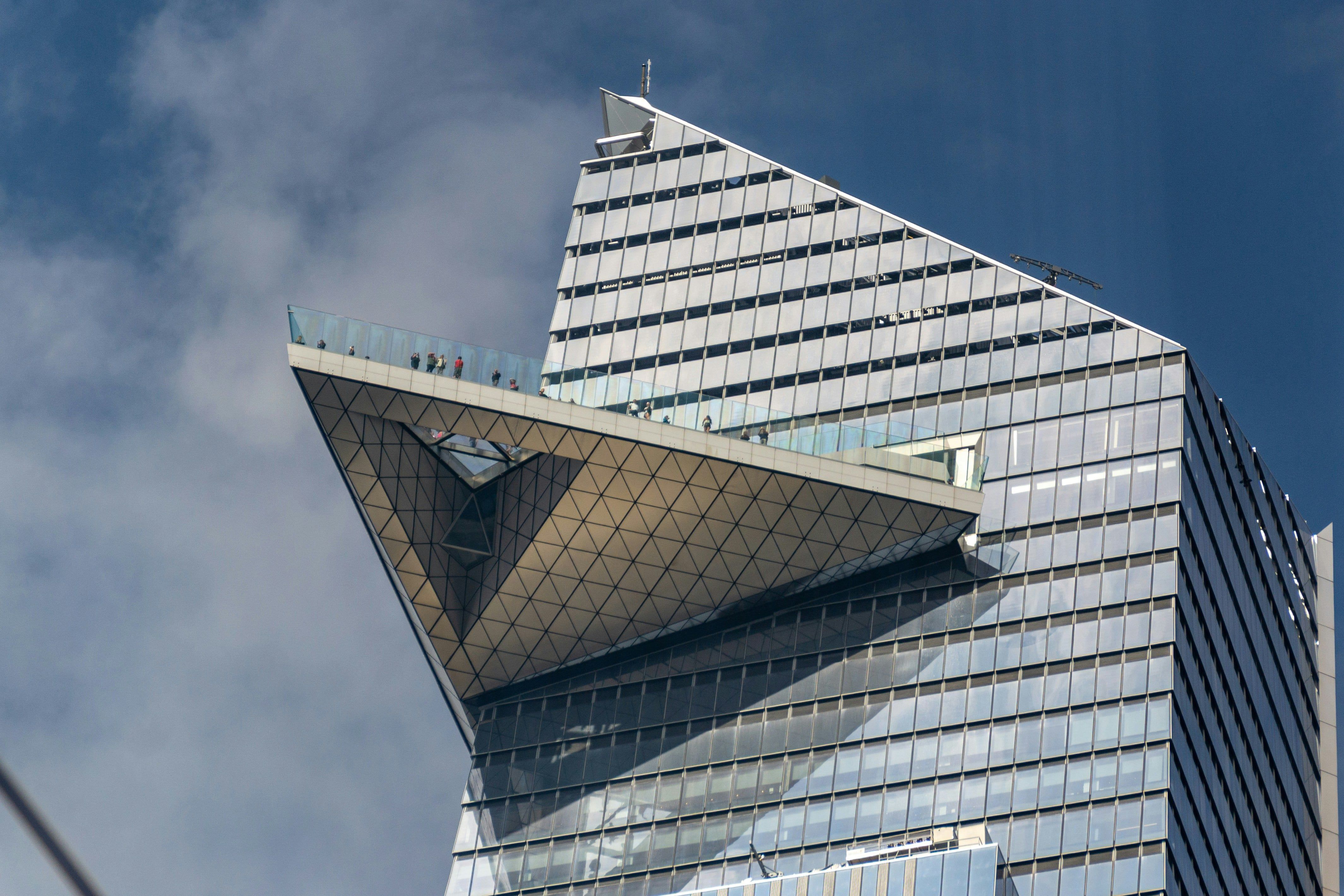
Adaptive Generative Models
What are Adaptive Generative Models?
Adaptive Generative Models are advanced AI systems that dynamically adjust their behavior to generate content or responses based on evolving contexts and user inputs. Unlike static generative models, these models learn and adapt over time, making them ideal for personalized applications, dynamic content creation, and problem-solving. They leverage architectures like transformers and reinforcement learning to continuously refine their outputs.
Why are they Important?
Adaptive Generative Models enhance the flexibility and relevance of AI systems by tailoring outputs to specific scenarios and users. This adaptability improves user experiences, boosts efficiency in various applications, and enables real-time learning from feedback. They are pivotal in areas requiring personalized interactions, such as customer support, creative tools, and education platforms.
How are they Managed and Where are they Used?
These models are managed by training on diverse datasets and incorporating feedback mechanisms to refine their outputs. They often use reinforcement learning with human feedback (RLHF) to align responses with desired outcomes. Adaptive Generative Models are widely used in:
- Personalized Content Generation: Creating tailored marketing copy or educational material.
- Customer Support Systems: Delivering context-aware and dynamic responses.
- Creative Applications: Producing adaptive designs, art, or music based on user preferences.
Key Elements
- Dynamic Context Understanding: Adjusts outputs based on changing input scenarios.
- Feedback Integration: Uses real-time feedback to refine content and responses.
- Reinforcement Learning: Optimizes performance through reward-based training.
- Personalization Capabilities: Adapts to individual user needs and preferences.
- Scalability: Handles diverse applications with varying complexity and context.
Recent Posts
Real-World Examples
- Chatbots: Adapting responses based on user interactions for more meaningful conversations.
- E-Commerce Platforms: Personalizing product recommendations and promotional offers.
- Creative Tools: Generating art, music, or design tailored to user preferences.
- Education Systems: Customizing learning materials to suit individual student needs.
- Healthcare Applications: Providing personalized patient care recommendations based on real-time data.
Use Cases
- Marketing Automation: Crafting personalized email campaigns and ad copy dynamically.
- Content Creation Platforms: Supporting writers with adaptive content suggestions.
- Virtual Assistants: Enhancing user experiences by adapting to individual preferences.
- Gaming: Generating interactive, evolving narratives and character dialogues.
- Financial Analysis: Producing tailored insights and reports for investors or clients.
Frequently Asked Questions (FAQs):
They are used to generate dynamic, context-aware content and responses, offering personalized solutions across industries like customer service and content creation.
Adaptive models evolve based on feedback and context, while static models produce fixed responses without considering changes in input or scenarios.
Industries like e-commerce, education, creative arts, and healthcare use these models for personalized and context-aware applications.
Their ability to incorporate feedback, personalize outputs, and dynamically adapt to evolving contexts sets them apart from traditional generative models.
Challenges include managing feedback systems, ensuring response accuracy, and balancing adaptability with computational efficiency.
Are You Ready to Make AI Work for You?
Simplify your AI journey with solutions that integrate seamlessly, empower your teams, and deliver real results. Jyn turns complexity into a clear path to success.